Synthetic data will dominate AI by 2025, growing from $381 million to $2.1 billion by 2028. It’s not just hype. Banks, hospitals, and autonomous vehicle companies are already using fake data that maintains real statistical patterns without privacy risks. Text-based synthetic data leads the pack, letting companies train models without expensive real data collection. Organizations slow to adopt might find themselves seriously disadvantaged. The synthetic revolution waits for no one.
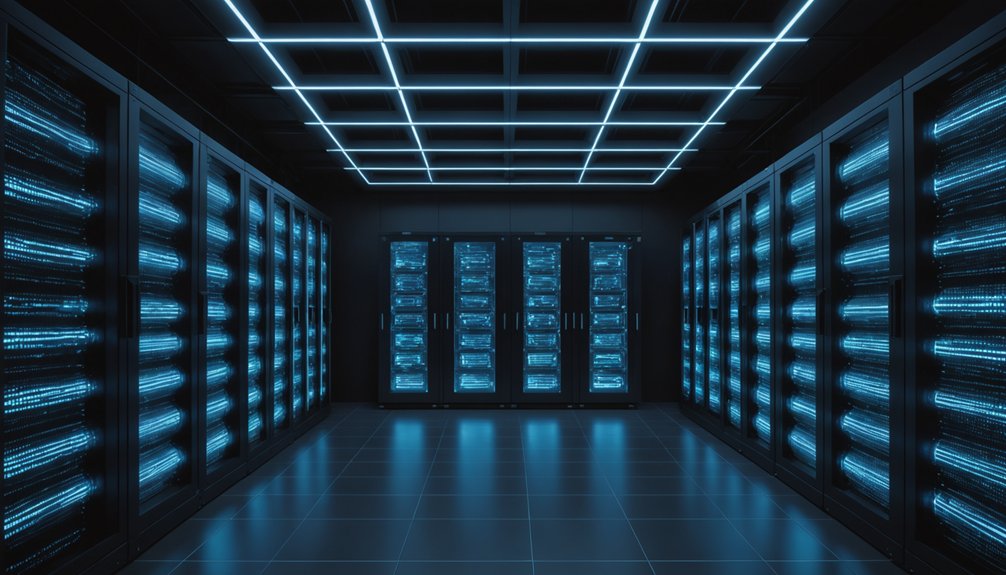
How did synthetic data become the backbone of AI innovation so quickly? Just a few years ago, real-world data dominated AI training. Now synthetic data is poised to overshadow it by 2030. The reason? It works. Really well.
Synthetic data—artificially generated information that mimics real-world data without actual personal details—has transformed from niche concept to industry necessity. By 2028, this market will hit $2.1 billion, up from a measly $381.3 million in 2022. That’s explosive growth. No wonder companies are scrambling to implement it.
The appeal is obvious. Privacy regulations are a nightmare for data scientists. Synthetic data eliminates the risk. No real customer information means no privacy violations. Simple as that. It’s expected to help organizations avoid up to 70% of privacy violation sanctions. That’s not just convenient—it’s financially critical.
Synthetic data isn’t just a privacy solution—it’s a financial lifesaver in today’s regulatory minefield.
Industries across the board are jumping on the synthetic data train. Banks use it to test fraud detection systems without exposing customer data. Similar to how diagnostic precision has improved in healthcare through AI algorithms, hospitals create fake patient records for diagnostic model training. Hospitals create fake patient records for diagnostic model training. Retailers optimize inventory with synthetic customer behavior. Even autonomous vehicle companies simulate dangerous driving scenarios without, you know, actual danger. Leading organizations now employ fairness audits to ensure synthetic datasets maintain equitable representation across all demographic groups.
The tools making this possible—K2view, Gretel, MOSTLY AI, Syntho, YData, Hazy—have evolved dramatically. They’re creating fake data so realistic it maintains statistical patterns of the original. Pretty impressive stuff.
For AI training, synthetic data is revolutionary. By 2030, over 95% of image and video training will use synthetic data. Real data collection is expensive and time-consuming. Synthetic data? Quick and cheap. Companies can generate exactly what they need, when they need it.
Small businesses benefit too. Data democratization means organizations without massive resources can still train sophisticated AI models. The playing field is leveling. This generative AI enhancement has dramatically improved the capabilities of synthetic data solutions, particularly in creating hyper-realistic text patterns that were previously difficult to simulate.
The future is clear. Synthetic data isn’t just a trend—it’s becoming fundamental infrastructure. Synthetic text-based data remains the most widely used type among organizations, allowing companies to simulate everything from customer reviews to technical documentation without privacy concerns. Organizations slow to adopt will find themselves at a serious competitive disadvantage. In 2025, that’s just reality.
Frequently Asked Questions
How Do Privacy Laws Impact Synthetic Data Usage?
Privacy laws create a complicated dance for synthetic data users.
GDPR and CCPA demand compliance through risk assessments and transparency. Companies can’t just generate data and call it a day. Re-identification risks remain real.
Smart organizations implement differential privacy techniques and anonymization methods to stay legal. Privacy-by-design isn’t optional anymore.
The upside? Synthetic data actually helps with data minimization requirements.
Still, continuous monitoring is essential. Privacy attacks happen.
Can Synthetic Data Fully Replace Real-World Datasets?
Synthetic data can’t fully replace real-world datasets—not yet, anyway.
Sure, it solves privacy headaches and scales beautifully, but it misses those weird edge cases that make reality… well, real. The quality varies wildly depending on generative models used.
For critical applications? Hybrid approaches work best.
Synthetic data handles volume and privacy concerns, while real data keeps things grounded in actual human behavior.
Perfect replacement? Nope. Valuable complement? Absolutely.
What Skills Are Needed to Implement Synthetic Data Solutions?
Implementing synthetic data solutions requires a multi-faceted skill set.
Math wizards who understand statistical modeling are essential—no way around it. Technical chops in generative AI models, particularly GANs and VAEs, separate the pros from the wannabes.
Data privacy knowledge? Non-negotiable. The field demands both technical expertise and business acumen.
Projects fail without proper integration skills and regulatory compliance understanding. And let’s be real—you’ll need troubleshooting abilities when things inevitably go sideways.
How Is Synthetic Data Quality Measured and Validated?
Synthetic data quality isn’t a guessing game. It’s measured through fidelity metrics like histogram similarity and correlation scores that compare synthetic data to real data.
Validation? That’s where the rubber meets the road. The TSTR method trains models on synthetic data and tests them on real data. Performance differences tell the truth.
Some frameworks like SynEval handle the heavy lifting, measuring everything from statistical similarities to privacy concerns. No shortcuts here.
What Are the Computational Costs of Generating Synthetic Data?
Generating synthetic data isn’t cheap. Period.
Computational costs stack up fast—cloud services charge by usage, dedicated hardware burns money, and intensive processing demands serious power. Companies shell out for high-end AI platforms, software licenses, and customization work.
Then there’s maintenance: server upkeep, algorithm updates, storage expenses. Quality validation? Another budget line.
The more complex and realistic the synthetic data needs to be, the deeper organizations dig into their pockets.