Computer vision is transforming healthcare in dramatic ways. Medical imaging analysis now spots diseases with superhuman accuracy. Early detection systems catch conditions before symptoms appear—saving lives, period. Surgeons perform with robot-assisted precision while pathologists identify cancer cells faster than ever. Specialized applications help with everything from retinal disease detection to monitoring elderly patients. The technology isn’t just impressive—it’s completely reshaping patient outcomes across specialties.
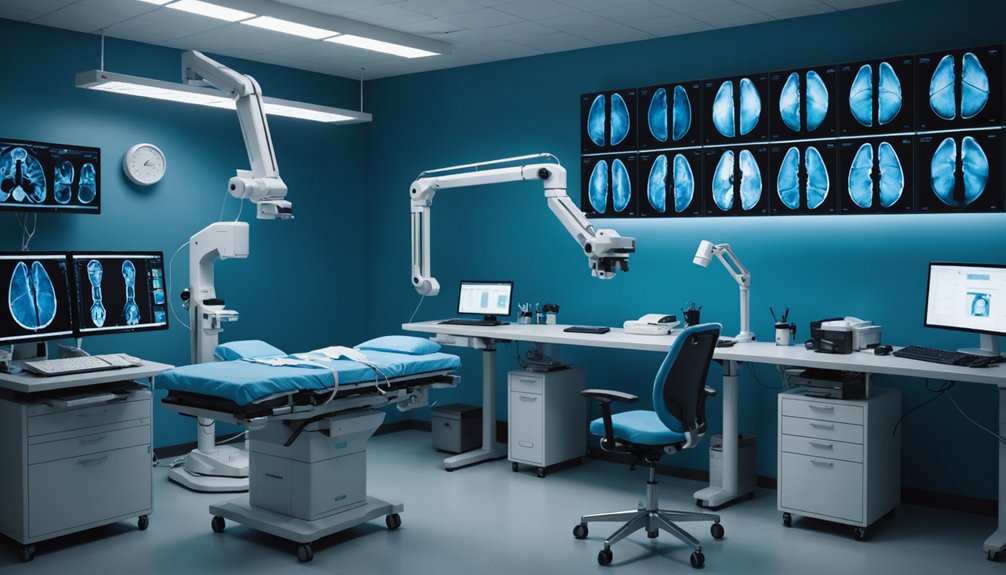
While doctors have relied on their eyes and expertise for centuries, computer vision is revolutionizing healthcare in ways nobody saw coming. Computers don’t get tired. They don’t miss details because they stayed up all night with a sick kid. They just see patterns—millions of them—faster than any human could hope to process.
Medical imaging analysis has gotten ridiculously good. These systems spot abnormalities in X-rays, MRIs, CT scans, and ultrasounds with accuracy that makes human radiologists do a double-take. They’re fast too. What might take a doctor hours to analyze, these machines handle in minutes. Less waiting, quicker treatment, better outcomes. Simple math. Studies show that diagnostic precision rates have significantly improved with AI-powered analysis compared to traditional methods.
Medical AI doesn’t just match human accuracy—it blows past it while we’re still loading the first scan.
Early disease detection is where things get interesting. Computer vision doesn’t just see what’s there—it sees what’s coming. Cancer, Alzheimer’s, and other conditions leave subtle fingerprints long before symptoms show up. AI spots these patterns. Patients live longer. End of story. Like autonomous vehicles using predictive modeling to prevent accidents, healthcare AI can anticipate potential health issues before they become critical.
Surgery has changed forever. Robot-assisted procedures guided by computer vision give surgeons superhuman precision. They can see inside bodies with clarity that was science fiction a generation ago. Difficult spots become accessible. Procedures get less invasive. Patients recover faster. Everyone wins.
In pathology labs across the world, computer vision examines tissues at a cellular level. Cancer cells hiding among thousands of normal ones? Found them. Complex tissue patterns that might confuse human eyes? Categorized and flagged. Pathologists now focus on tough cases while algorithms handle routine work.
Ophthalmologists are using these systems to detect retinal diseases that once required specialized equipment and expertise. A simple scan, analyzed by computer vision, can spot trouble brewing before vision loss begins.
The future is clear: medicine will never be the same. Computer vision doesn’t replace doctors—it makes them better. Patients get diagnosed sooner, treated more precisely, and recover faster. In dermatology, these technologies can identify visual indicators of skin conditions and provide personalized skincare recommendations based on comprehensive image analysis. Not bad for a bunch of algorithms that just learned how to see.
For elderly individuals living independently, computer vision technology has become a lifeline through fall detection systems that monitor movements and automatically alert caregivers when accidents happen.
Frequently Asked Questions
What Privacy Regulations Affect Computer Vision in Healthcare?
Computer vision in healthcare faces serious regulatory hurdles. HIPAA is the big one in the U.S., protecting patient data with strict rules.
GDPR hits even harder in Europe. Don’t forget CPRA in California and PHIPA in Canada. These laws aren’t messing around.
Medical images contain sensitive personal information—can’t just throw those around. Providers need encryption, access controls, and audit trails to stay compliant.
New tech like federated learning helps keep the privacy police at bay.
How Accurate Is Computer Vision Compared to Human Diagnosticians?
Computer vision often matches or exceeds human diagnosticians in accuracy, especially for pattern recognition tasks.
It excels at detecting subtle anomalies humans might miss, like early-stage cancers or hairline fractures. Unlike humans, these systems never get tired – no coffee breaks needed!
However, they lack clinical judgment and contextual understanding. The real magic happens when both work together – computer vision‘s consistency paired with a doctor’s experience. A pretty unbeatable combo, honestly.
What Training Data Requirements Exist for Medical Vision Systems?
Medical vision systems demand specific training data requirements. High-resolution, diverse images are non-negotiable. Period.
They need tons of properly annotated examples across demographics—age, gender, race. The catch? Medical experts must do the labeling, which isn’t cheap or quick.
Quality matters too. No distorted images allowed. Only authentic, unaltered visuals make the cut.
Systems integrated into healthcare settings need regular dataset updates to stay relevant.
Training data quantity directly impacts reliability. More data, better results. Simple as that.
How Do Healthcare Professionals Adapt to Computer Vision Technology?
Healthcare professionals adapt to computer vision through structured training programs. They learn to interpret AI-generated results, integrate new workflows, and maintain oversight of the technology.
It’s not easy. Resistance happens. Some docs just don’t trust machines.
Successful adaptation requires continuous education, collaborative implementation, and gradual integration rather than abrupt changes.
Clinical champions who advocate for these technologies help bridge the gap between technical capabilities and practical application.
Critical thinking remains essential.
What Are the Implementation Costs of Medical Computer Vision Systems?
Medical computer vision systems aren’t cheap. Basic AI vision software starts around $30,000, while complex systems jump to $90,000+.
Development takes 6-12 months and advanced healthcare applications can reach $400,000+.
Don’t forget infrastructure costs—up to $1 million annually. Customization adds 30-40% to the price tag.
Cloud deployment might save some cash, but regulatory compliance? That’s another expense.
Legacy system upgrades? Tack on another 20-30%.
Healthcare innovation comes with a hefty invoice.