AI-driven predictive maintenance isn’t revolutionary in 2025, just maturing. Growing from $0.88 billion to $1.02 billion at 15.7%, it’s evolution, not revolution. Manufacturing plants love it—who wouldn’t? It cuts costs by 30% and prevents those profit-killing breakdowns. Deep learning spots subtle problems human eyes miss. But garbage data equals garbage predictions, no magic here. The real breakthrough? Companies finally figuring out how to implement it properly.
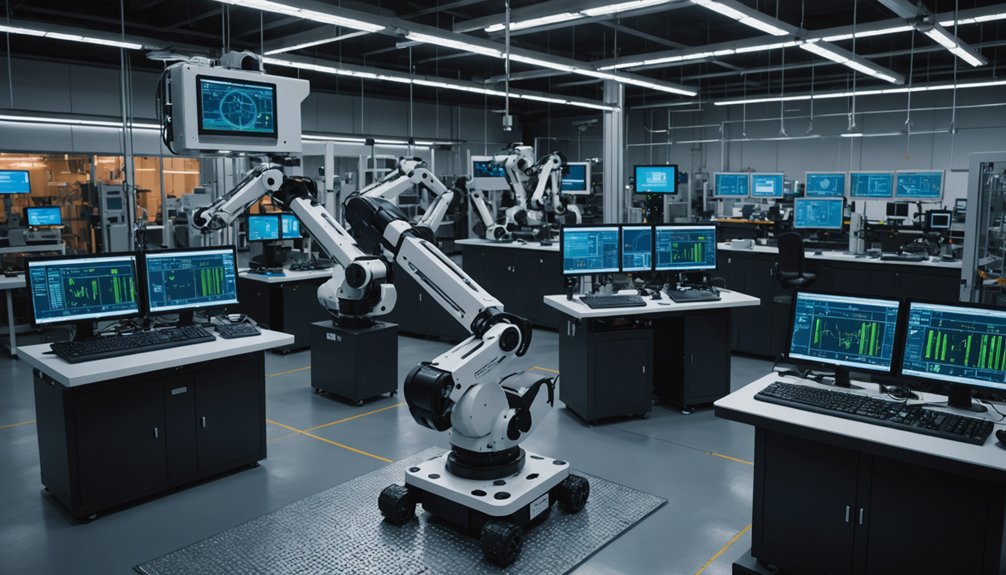
While companies have been talking about predictive maintenance for years, AI is finally turning this pipe dream into cold, hard reality. The numbers don’t lie – a market worth $0.88 billion in 2024 is jumping to $1.02 billion in 2025. That’s a 15.7% growth rate. Not too shabby for technology that fundamentally tells you when your expensive equipment is about to throw a tantrum.
Let’s be real. Equipment fails. Always has, always will. But now, instead of waiting for catastrophic breakdowns that cost millions, AI algorithms analyze data patterns and essentially whisper, “Hey, this thing’s gonna break in about three days.” Machine learning models crunch through mountains of sensor data, spotting the subtle warning signs humans miss. It’s like having a psychic mechanic, minus the crystal ball and incense. Similar to how predictive analytics revolutionize supply chain operations, these AI systems are transforming equipment maintenance.
The manufacturing sector is eating this up. Power plants too. Why? Because unplanned downtime is a profit-killer. When a production line goes down unexpectedly, executives lose their minds – and their quarterly bonuses. AI-powered predictive maintenance means fewer emergency repairs, fewer replacement parts, and fewer middle-of-the-night panic calls. This approach has demonstrated up to 30% cost reduction in maintenance operations while extending equipment life. The technology represents a significant shift from reactive maintenance strategies to more proactive approaches that anticipate failures before they occur.
Deep learning has supercharged these capabilities. The technology doesn’t just spot obvious problems; it finds complex patterns that indicate future failures. Much like diagnostic precision in healthcare, these systems can detect subtle anomalies that humans might overlook. Real-time monitoring means issues get flagged immediately, not after something’s already smoking and sparking.
Of course, it’s not magic. Garbage data in, garbage predictions out. Companies investing in this technology need clean, consistent data streams. But those who get it right are seeing serious operational efficiency gains.
Is it revolutionary? Maybe that’s overstating things. Evolution rather than revolution. But when you’re saving millions by fixing small problems before they become catastrophes, does it really matter what you call it? For industries from automotive to aerospace to healthcare, the answer is clearly no. They’re too busy counting their savings.
Frequently Asked Questions
How Much Do AI Predictive Maintenance Solutions Typically Cost?
AI predictive maintenance costs vary widely. Initial investment typically absorbs 20% of maintenance budgets, with another 15% for training staff. That’s a chunk of change.
Some vendors like Guidewheel offer solutions without extra hardware requirements—definitely more affordable. Companies pay for the promise: 70% reduction in equipment failures and 25% boost in productivity.
Worth it? Depends on your downtime costs. The market’s growing fast regardless.
Can AI Predictive Maintenance Be Integrated With Legacy Systems?
Yes, AI predictive maintenance can be integrated with legacy systems.
It’s not always pretty though. Older equipment needs IoT sensors retrofitted to capture essential data. Companies must standardize data formats—a real headache with decades-old machinery.
Cloud computing bridges the gap between ancient hardware and modern algorithms. Staff training is vital too.
Despite challenges, the benefits are clear: less downtime, better efficiency, and squeezing more life from aging infrastructure.
What Security Risks Come With Implementing AI Predictive Maintenance?
AI predictive maintenance brings serious security baggage. Data leaks happen. Systems get hacked. Insiders with access can wreak havoc. Network vulnerabilities? Everywhere.
The threats get technical too. Data poisoning corrupts AI models. Prompt injection attacks manipulate outputs. And good luck with transparency—these systems are black boxes.
Companies need encryption, access controls, and network segmentation. Regular audits aren’t optional. They’re survival tools.
Which Industries See the Lowest ROI From Predictive Maintenance?
Industries with the lowest ROI from predictive maintenance?
Small-scale manufacturing tops the list. They’re sinking big costs into systems that barely justify themselves.
Non-critical asset management comes next—why predict failures for stuff that barely matters?
Low-volume production facilities get minimal returns too.
Places with ancient, mismatched equipment struggle to see benefits.
And companies with simple operations? They’re basically buying a Ferrari to drive to the corner store.
How Long Does Implementation of AI Predictive Maintenance Typically Take?
Implementation of AI predictive maintenance isn’t quick. Companies should expect a timeline of 6-18 months.
The journey has distinct phases: data collection (several weeks), AI model development (a few weeks to months), and system integration (months to a year).
Each phase has its challenges. Larger facilities with complex equipment? Longer timelines.
Smaller operations with existing data infrastructure? Faster deployment.
No shortcuts here.