A/B testing eliminates business guesswork in three steps. First, select one impactful element to test—like a CTA button or headline. Second, establish clear objectives with a measurable hypothesis (e.g., “red buttons will increase clicks by 15%”). Third, run the test by randomly splitting your audience, then analyze the statistical significance of results. No gut feelings allowed. Data drives decisions, not hunches. The difference between gambling and strategy? It’s all in the testing.
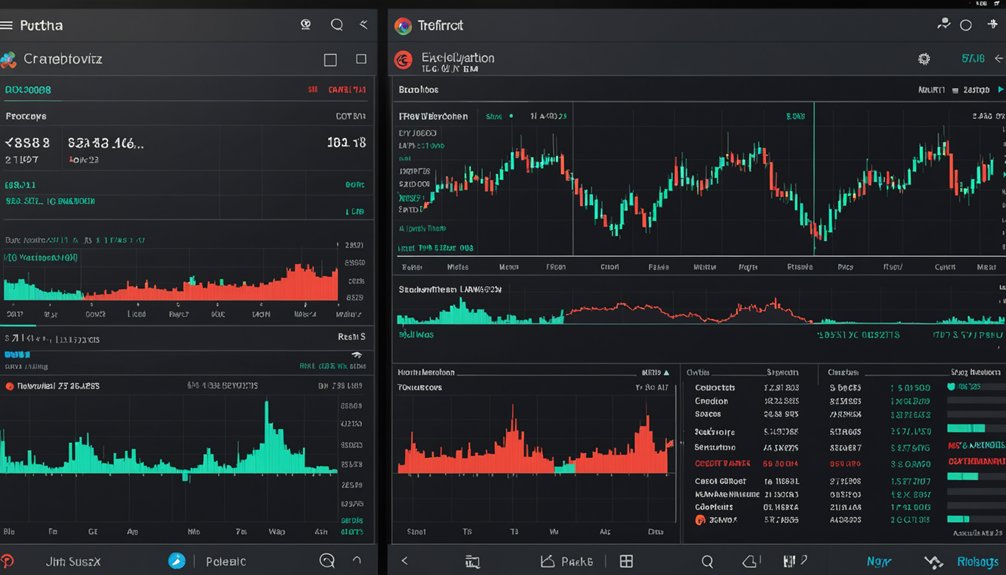
When businesses make decisions based on gut feelings, they’re basically just gambling with their success. A/B testing changes that. It’s a method that compares two versions of something—maybe a webpage, an email subject line, or that big red “BUY NOW” button everyone seems to love. Real data. Real results. No more guessing.
Gut feelings are business gambling. A/B testing brings real data to replace wild guesses.
First, choose what to test. This sounds obvious, but you’d be shocked how many companies try testing everything at once. Bad move. Pick one element—just one. Maybe it’s that CTA button color or the headline on your landing page. Whatever it is, make sure it matters to your bottom line. Testing the font on your privacy policy? Waste of time. Nobody reads that anyway.
Next, set clear objectives and create a hypothesis. What exactly are you trying to improve? More newsletter sign-ups? Higher conversion rates? Better engagement? Your test needs a purpose. And not some vague “make things better” nonsense. Get specific. “We believe changing our button from blue to red will increase clicks by 15%.” That’s a hypothesis with teeth. Modern machine learning algorithms now enhance hypothesis generation by analyzing past campaign performance data.
Finally, run the test properly. Split your audience randomly—emphasis on randomly. Use proper tools like Optimizely or VWO instead of some homemade spreadsheet contraption. Popular platforms like Google Optimize can automate your testing process for more reliable results. Let the test run long enough to gather meaningful data. A day or two won’t cut it. Remember that responses may vary significantly among different customer segments, requiring you to analyze results accordingly. Pre-test research using existing data can significantly enhance the relevance and effectiveness of your experiments.
And for heaven’s sake, only change one thing between versions. Change multiple elements and you’ll have no idea what actually worked.
After collecting data, analyze the results with statistical testing. Numbers don’t lie, but they can mislead if you don’t understand significance. Did version B really win, or was it just luck? Document everything. Learn from it. Implement what works.
A/B testing isn’t rocket science. But it’s not finger painting either. Do it right, and you’ll make decisions based on facts, not feelings. Your wallet will thank you.
Frequently Asked Questions
How Long Should an A/B Test Run?
A/B tests typically should run for at least 2 weeks.
Why? Because data doesn’t lie, but it sure can mislead if you rush it. Statistical significance matters, folks. Daily and weekly variations need to be accounted for – Monday shoppers aren’t Friday browsers.
Sample size is critical. More traffic? Maybe shorter tests work. Lower volume sites? Buckle up for the long haul.
And please, resist the urge to call it quits when early results look good. Patience pays off.
What Sample Size Is Needed for Statistical Significance?
Sample size for statistical significance depends on several key factors.
Effect size, significance level (usually 0.05), statistical power (often 0.8), and baseline conversion rate all matter here. Too small? Results become unreliable. Too large? Waste of resources.
Online calculators simplify this complex math. No universal number exists—it’s all relative to your specific situation.
Most tests aim for 95% confidence level. Bottom line: proper sizing prevents false conclusions and strengthens stakeholder confidence. Math isn’t sexy, but it’s necessary.
Can I Test Multiple Variables Simultaneously?
Yes, you can test multiple variables simultaneously. It’s called multivariate testing.
Different from standard A/B tests. Requires larger sample sizes though – that’s the catch. Great for understanding how elements interact with each other.
Not ideal for low-traffic sites. Some marketers prefer running separate A/B tests to avoid complications.
Alternatively, you could use mutually exclusive experiences to run multiple tests without overlap.
Complex? Sometimes. Effective? Often. Worth the effort? Depends on your traffic.
How Do I Handle Seasonal Fluctuations in A/B Testing?
Handling seasonal fluctuations in A/B testing requires careful planning.
Marketers should identify predictable seasonal trends and adapt their testing strategies accordingly. Christmas, Easter, Super Bowl – they all change how people shop.
Smart testers account for these external factors by adjusting test durations and sample sizes. Traffic spikes during holidays? Perfect time to validate hypotheses quickly.
The key is recognizing that seasonal data isn’t an anomaly – it’s an opportunity. Use it. Optimize for it. Your competitors certainly are.
Should I Inform Users They’re Participating in A/B Tests?
Informing users about A/B tests is tricky. Not telling them prevents behavior changes that could skew results. But transparency builds trust. Sometimes, it’s legally required anyway.
Industry practice varies. Most companies don’t explicitly announce every test. They include testing in their terms of service instead.
Bottom line: If the test involves sensitive data or significant experience changes, disclosure might be appropriate. Otherwise? Most companies just test quietly and move on.